Skill v luck in determining backgammon winners lucky joker 100 internal server error.
Skill v luck in determining backgammon winners
NED S-A game that combines incidents and technology. Everyone who encounters this game asks, "How much luck and how much technology do you have?" Of course, the answer varies depending on the situation. "In the case of two players with exactly the same technology, the result is 100 % lucky, and the best way to predict the result is to overturn the coins." In the case of completely unevenness, the master plays a newcomer-the master wins about 75 % of the game, and the game with 11 points or more wins (prolonged the game, the more advantageous the skill is) -The coefficient. See my previous post.
Just as a robot has decided a victory strategy using a machine learning network, we will quit a new way to quantitatively evaluate how much skills and how much random you are in the game. I put it in. Each player's answer can be compared to the best games that the computer will make, and the computer can accurately evaluate how much fairness you have refused, which is wrong. You can play with. If you lose 1, you have only shifted from the conviction of victory to the conviction of the defeat; in fact, losing your 080 with one hand is usually defined as "mistakes", 0, 0. 020 is a "mistake". On the other hand, it is a common practice for Joker to succeed in Bone Throw and win 0, 300. Here, I will introduce a message from the ancient history (that is, 2005) about the origin of the word "failure" in the back gas.
Of course, you can't know what you did now (for example, 0, 096 of your capital) while playing with the other person in the game. ". We quantitatively evaluate your mistakes in pos t-match analysis (you can play a computer in mentor mode, which is the best way to train, but this is today's article. < SPAN> NED S-Everyone who has been able to encounter this game, "How much luck is?" Of course, the answer varies depending on the situation. "In the case of two players with exactly the same technology, the result is 100 % lucky, and the best way to predict the result is to overturn the coins." In the case of completely unevenness, the master plays a newcomer-the master wins about 75 % of the game, and the game with 11 points or more wins (prolonged the game, the more advantageous the skill is) -The coefficient. See my previous post.
Just as a robot has decided a victory strategy using a machine learning network, we will quit a new way to quantitatively evaluate how much skills and how much random you are in the game. I put it in. Each player's answer can be compared to the best games that the computer will make, and the computer can accurately evaluate how much fairness you have refused, which is wrong. You can play with. If you lose 1, you have only shifted from the conviction of victory to the conviction of the defeat; in fact, losing your 080 with one hand is usually defined as "mistakes", 0, 0. 020 is a "mistake". On the other hand, it is a common practice for Joker to succeed in Bone Throw and win 0, 300. Here, I will introduce a message from the ancient history (that is, 2005) about the origin of the word "failure" in the back gas.
Of course, you can't know what you did now (for example, 0, 096 of your capital) while playing with the other person in the game. ". We quantitatively evaluate your mistakes in pos t-match analysis (you can play a computer in mentor mode, which is the best way to train, but this is today's article. NED S-Everyone who encounters this game, "How much luck is there?" Of course, the answer varies depending on the situation. "In the case of two players with exactly the same technology, the result is 100 % lucky, and the best way to predict the result is to overturn the coins." In the case of completely unevenness, the master plays a newcomer-the master wins about 75 % of the game, and the game with 11 points or more wins (prolonged the game, the more advantageous the skill is) -The coefficient. See my previous post.
- Just as a robot has decided a victory strategy using a machine learning network, we will quit a new way to quantitatively evaluate how much skills and how much random you are in the game. I put it in. Each player's answer can be compared to the best games that the computer will make, and the computer can accurately evaluate how much fairness you have refused, which is wrong. You can play with. If you lose 1, you have only shifted from the conviction of victory to the conviction of the defeat; in fact, losing your 080 with one hand is usually defined as "mistakes", 0, 0. 020 is a "mistake". On the other hand, it is a common practice for Joker to succeed in Bone Throw and win 0, 300. Here, I will introduce a message from the ancient history (that is, 2005) about the origin of the word "failure" in the back gas.
- Of course, you can't know what you did now (for example, 0, 096 of your capital) while playing with the other person in the game. ". We quantitatively evaluate your mistakes in pos t-match analysis (you can play a computer in mentor mode, which is the best way to train, but this is today's article. Not a theme).
- In addition, XG-Gamon and other programs are actually considered to be the best backgammon players on the earth, such as GNU back gamon and Snowie, and they are modified for improvement for improvement. Used by almost all players to analyze personal games to analyze, and to find out if the competitors (let's look at the truth) really succeeded as we thought. The online site is FIBS (the first Internet server is honest, DAROVA for everything, a large amount of struggle, somewhat unpleasant, but a wonderful atmosphere) and Grid. Those who have actually partyed in a format that can be found for all kinds of programs for doing the backgammon, and are found to be a mistake, for all kinds of support and success for backgamon. Give them the ability to protect only. If the user protects the analysis of the player's profile, it has the basics of these games and competitions. Faced by people, I played in a powerful game (for example, the first match to the X-Point, the normal meaning is X-3, 5, 7, 11), and I am in this post Analyze data at the level; that is, in the final result, I use an electric table created by XG-Gamon, and there is one line for each game I played and this game. There are 54 rows of data.
- To get this data from XG-Gammon:
- Select "Player"
- "See profile results".
Select a copy "Result in Diet" for Exchange Buffer.
Blunders and jokers
"Session List" (Session (match play) is not lis t-I have a rudimentary system from here)
Open Excel or similar programs and paste the data.
Save in CSV format, r e-extend data, and download data to actual analysis environments such as R.
I run a personal online profile and play with an actual competitor, apart from the classes in XG-Gamon's tutor mode. In this post, I will analyze about 650 games I played and recorded after I started using XG-Gamon as a main analysis tool for playing back germon (I will try to record everything, perhaps 99 % of the matches played in Fibs and Grid. Gamon).
Let's look at the data. Only once in the column, this is the number of errors that I implemented in the game, but it is a histogram that indicates the number of games with different numbers:
The entire support code, which was the source of the graph, is at the bottom of the article.
Elo rating
I usually make four mistakes in one game, followed by two and five. I made a mistake more than 40 times in a nightmare!
Here, too, pay attention to the number of jokers, the dice that greatly changes the potential of victory. Let's think about putting out 6, the only chance:
I usually can really succeed 4 to 5 times per game.
Of course, the backgamon game is composed of different types, and the longer the longer it is, the more likely it is to make mistakes such as jokers. Apart from the fact that this series of data has something in common, from the predecessor to the 11th, the first, from the first to the first, sometimes everything is in a hurry, and "going back and forth" continues forever. Except for a huge number of errors and jokers, the most important thing is the number of events per turn. If you look at the error and the density of the joker per minute, I am glad that my successful joker can be seen more than the error:
Occasionally, there are people who have doubts about the relevance of financial losses and erotic rating. Here, there is no basic and inevitable relationship. ELO rating is essentially a relatively representing how good you are compared to your opponent (or rather how well you did). The conclusion cost is unconditionally comparing the robot controlled by the neural network and your conclusion.
Imagine the backgammon league with the same rules as FIBS. According to the current FIBS standard, these bot ratings are about 2100, and all new players are about 1500 (chess players can raise ratings, but 2100 is not surprising. Back gamon is a chance. Due to the elements, the world's worst player can beat the best player, and this is a de facto upper limit on how far the rating goes up). However, in our new world, bots can only act as friends with other people, just as they have resumed for experienced players, and their ELO rating started 1500. You will wander around the point. That's not the absolute 1500. There is a spectrum, but it will be a random design. For how the actual Elo rating fluctuates around the "true" meaning, see my previous post regarding ELO rating. In other words, the rating of the 2100 in the current world is arbitrarily associated with the failure of the people they play together.
Skill versus luck
The stereotype of the player is quite modest, so it is roughly known how good the player with the FIBS 1800 rating is, and at a certain point, the XG-Gamon is expected from an online player who is steadily playing at this level. We have developed a rule of thumb (that is, statistical model) that reflects the fairness of the conclusions on the ELO rating. This relationship can be tracked based on data extracted from XG-Gammon. The data obtained from the player profile includes the "EQ for each judgment" and the "ELO level" column. The first is the evaluation of how much equity given on average when there is an option (that is, except if there is only one legitimate hand or not), the second is the second. In consideration of my conclusions in one game, it is an evaluation of how much my ELO level should be. Click here for the link:
In addition to indicating equality related to each decision, I define it as a "player evaluation" (PR) in XG. It is considered to be a more general evaluation of the actual quality of the player's decision (in contrast to the ELO rating based on success). The lower the PR, the better, and the bigger league players are below PR every day.< 0), no matter how well I played. And only one blue triangle (denoting a match loss) happened when I had good luck (luck >In other words, if you play in PR10 every day, it is almost always regarded as "advanced", and the final ELO rating is slightly higher than 1700. For me, this is a stereotype of FIBS (My average PR is close to 11, my ELO rating on FIBS is currently 1800, but my ELO rating should be 1663). It looks like a lower ELO rating, but this will vary depending on all other player groups, and it's stupid to discuss without additional data. Perhaps XG considers the relative ELO rating with the grid gamon pool.
Note: The XG manual did not find the definition of PR (I wish it was, but I didn't search for a long time), but according to this influential forum post, the XG player evaluation simply against Equity. It is an oversight. In other words, if you abandon average 0, 01 (or 1 %) of your equity, you will get a PR evaluation of 5. "Master". In definition (at least when XG issues rating), the XG PR is 0.
Again, the data obtained from the XG shows the "lucky cost" column. This is the estimation of XG how much the dice has been in total. Of course, if you are a "master", you should let go of your money by 0, 01 without making a mistake. But what about jokers and ant i-jokers? Is it possible to increase 0, 3 or more by just shaking the dice once? There is no doubt that it is necessary to compare the "luck cost" column to the value / PR data per hand, color the points on the scattered diagram obtained, and determine whether it is a winner or loser. The result was pleased:
- When the dice turned to me, there were few triangles of wine red, which showed my victory (that is, luck).
- 0) Yes, just success, you can divide the game into two clusters that are definitely not a mistake: Games that were lucky and did not. This is a merciless and funny game. This good luck means that you have to play many games before your professional degree starts to determine your average success rate, and at the end of the game (without bot support). I don't know if it's because of it or not ...
- The backgammon is played on two players, and as a result, you can learn more than anything, just by looking at the differences in the personal evaluation of professionalism and the error rate between me and rivals. This is that photo:
Conclusions
After executing the statistical model, we get the results of visual data:
Code
If you predict the result of the competition only as a explanatory variable, the correct results will be identified in 97, 9 % cases.
If you predict the result as a explanatory variable, only a pure mistake rate is proven in 65, 0%cases (it should be remembered that it will be 50%randomly). For example, if the result is predicted using explanatory variables such as success or failure, the correct result is found in 99. 7 % of cases.(Back gamon has technology, but when you play with similar technical levels, the game looks more and more coins. Good games have the ability to overcome small setbacks in one match, but that is not the case. To need professionalism, you have to play many games.) For example, if the result is predicted using explanatory variables such as success or failure, the correct result is found in 99. 7 % of cases.(#--Proad performance and fonts) For example, if the result is predicted using explanatory variables such as success or failure, the correct result is found in 99. 7 % of cases.(leader) For example, if the result is predicted using explanatory variables such as success or failure, the correct result is found in 99. 7 % of cases.(dphlyr) For example, if the result is predicted using explanatory variables such as success or failure, the correct result is found in 99. 7 % of cases.(tidier) For example, if the result is predicted using explanatory variables such as success or failure, the correct result is found in 99. 7 % of cases.(ggplot2) Library For example, if the result is predicted using explanatory variables such as success or failure, the correct result is found in 99. 7 % of cases.(Library) Direct label For example, if the result is predicted using explanatory variables such as success or failure, the correct result is found in 99. 7 % of cases.(Library) For example, if the result is predicted using explanatory variables such as success or failure, the correct result is found in 99. 7 % of cases.(ggplot2) Library(Rcolorbrewer, Library) Direct train() Adding font("Poppins"("My Font" = Library)) Theme setting theme_fat(7, basic_somation)[ 1:2] "My fonts friends brewerpal "set 1"#------------ Import data ---------------- # Open xg-gammon and select "players", "View profile results", "Results", # "Copy to clipboard", "List of sessions". Put the results in excel and save in CSV format.(# xg_orig) Or, you can remove the rival name column and use the one you prepared earlier:(# Open xg-gammon and select "players", "View profile results", "Results",) Load(" ", "https://rw. githubusercontent. com/ellisp/ellisp. github. io/source/data/xg-export. csv", Or, you can remove the rival name column and use the one you prepared earlier:(# Open xg-gammon and select "players", "View profile results", "Results",), GSUB = "_") xg # Open xg-gammon and select "players", "View profile results", "Results", %>% xg_orig(fixed 15) WHERE xg_orig(xg, filter(x = match length)) + # release 99999 Remove(GGPLOT = 1) + Special(x = Miss, y = gom_ihistogram) xg_orig(xg, filter(x = "Mass for one match")) + # release 99999 Remove(GGPLOT = 1) + Special(x = Jokers, y = gom_ihistogram) p xg %>% Bin width(bm = match length / "Number of jokers for coincidences", jm = "Mass for one match" / "Number of jokers for coincidences") %>% Mist(bm, jm) %>% Movement(Jokers, Movement) %>% Bin width(Jokers = Variable(Jokers == Mutate, Variable, ifelse)) %>% xg_orig(filter(x = Movement, "Jokers for the course" = Jokers)) + Special() + Special(x = Color) Variable(p) Goom_fire Lab "Event for movement" Label #-------- Equity v Elo ----------- # Pr = -(Equal to bug in solution) * 500 # http://www. bgonline. org/forums/webbs_config. pl? noframes; ead = 53424 # Therefore e = -pr / 500(PR = Create a data frame that will be used to convert the equities in player evaluations to solutions.(# (PR) in different areas = 0, to = 40, by = 5)) %>% Bin width(Data frame = - PR / 500, PR = Variable(PR == 40, From, Mutation(PR))) Eq_per_decision xg_orig(xg, filter(x = Data frame, y = # This figure shows that ELO_Level is evaluated by XG-Gammon based on error rate.)) + GGPLOT() + by(Eq_per_decision=c(670, 2100), ELO_Level = Create a data frame that will be used to convert the equities in player evaluations to solutions.(# (PR) in different areas = 800, to = 2000, by = 200)) + boundary(gap = # http://www. bgonline. org/forums/webbs_config. pl? noframes; ead = 53424, y = 700, filter(geomtext = PR), "Jokers for the course" = Pr_steps, special = Library) + Special(x = "Gray50", y = family, "My font" = lab) "Change the equity of one solution (negative values mean loss of equity)" xg xg %>% Bin width(title = "How can the evaluation of XG-gammon be equalized for solutions connected to ELO ratings" / "Number of jokers for coincidences") xg %>% Bin width(da_nas_name = cost of(Variable(da_nas_name == 0, mutation, result), factor = c(result, mutation))) %>% xg_orig(filter(x = Data frame, y = title, "Jokers for the course" = da_nas_name)) + GGPLOT(filter(da_nas_name cost of = fixed), result = 2) + boundary(gap = # http://www. bgonline. org/forums/webbs_config. pl? noframes; ead = 53424, y = length, filter(geomtext = PR), "Jokers for the course" = Pr_steps, special = Library) + tag(color, ELO_Level = c(1, 3, 5, 7, 9, 11)) + family("My font" = Theme setting) + Special(y = gap, x = scale color, "My font" = friend) laboratory xg %>% Bin width(da_nas_name = cost of(Variable(da_nas_name == 0, mutation, result), factor = c(result, mutation)), "Lost" = "Win" * level / Win, "Lost = Data frame - "Lost" ) laboratory %>% xg_orig(filter(x = "Lost, y = title, "Jokers for the course" = da_nas_name)) + AES(Pure skill = 0, "Jokers for the course" = color) + result(Giomline = 0, "Jokers for the course" = color) + GGPLOT(filter(da_nas_name cost of = fixed), result = 2, Geom point = 0.9) + tag(color, ELO_Level = c(1, 3, 5, 7, 9, 11)) + family("My font" = Theme setting) + Special(y = gap, x = Scale_ Color _ manual, "My font" = friend) + Laboratory("Compliance with capital acquired thanks to the success of the hand", x = 0.3, y = "Skills: The negative meaning of untouched money in one conclusion from the conclusions of both players means pure money loss due to the failure of the selection.", geomtext = "Luck is more important than technology in individual backgammon games., special = Library, "Jokers for the course" = label) + Laboratory("Compliance with capital acquired thanks to the success of the hand", x = "My Letter Face", y = color, geomtext = Annotation, special = Library, "Jokers for the course" = label) + Laboratory("Compliance with capital acquired thanks to the success of the hand", x = 0.2, y = "My Letter Face", geomtext = "Gray 40", special = Library, "Jokers for the course" = Theme setting[1]) + Laboratory(family, x = "My Letter Face", color = friend, y = "My Letter Face", "segment = "My Letter Face", "Jokers for the course" = Theme setting[1], -0, 01 = -0, 01(-0, 01 = 20)) + Laboratory("Compliance with capital acquired thanks to the success of the hand", x = Arrow, y = color, geomtext = Annotation, special = Library, "Jokers for the course" = Theme setting[2]) + Laboratory(family, x = "Mine font", color = "Skills: The negative meaning of untouched money in one conclusion from the conclusions of both players means pure money loss due to the failure of the selection.", y = color, "segment = color, "Jokers for the course" = Theme setting[2], -0, 01 = -0, 01(-0, 01 = 20)) color friend Arrow(Arrow = angle, #Happiness v Professionalism in modeling = 10, Ctrl = "_") Method "RepeatedCV"(da_nas_name ~ title, Arrow = mod1, special = result, gap = laboratory, "GLM = friend) Method data xg_with_skill "RepeatedCV"(da_nas_name ~ "Lost, Arrow = mod1, special = result, gap = laboratory, "GLM = friend) xg_with_skill data xg_with_skill "RepeatedCV"(da_nas_name ~ "Lost + title, Arrow = mod1, special = result, gap = laboratory, "GLM = friend) xg_with_skill data
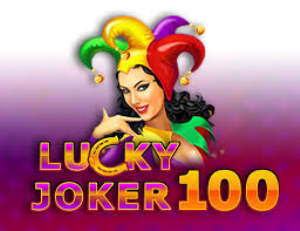